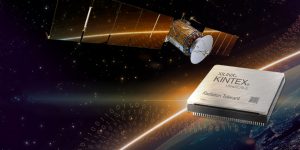
One of the major challenges in the space industry is the architecture of components used in various projects, whether they are global satellites or rockets to the Moon or Mars, is the fixed architecture which is designed for a specific mission, said Minal Sawant, system architect for Xilinx space products. A flexible architecture platform that can be used for multiple missions is one challenge, she told Electronics Weekly. “Learning and processing data in space is nascent,” she said. “We will see a shift towards machine learning (ML) and thing being done in space related to machine learning,” she added. ML is used for scientific analysis, object detection and image classification. Improving processing efficiency will reduce latency in operations both in orbit and on the ground.
This increased activity will require reliable components but also ones that adhere to the principles of size, weight and power (SWaP) and cost. The company has introduced the 20nm radiation tolerant (RT) Kintex UltraScale XQRKU060 FPGA which provides unlimited on-orbit reconfiguration with a 10-fold increase in DSP to increase throughput and bandwidth across all orbits and deep space.
The architecture features a design for single event effects (SEE) mitigation for use across low earth orbits (LEO), medium earth orbits (MEO), geosynchronous and deep space orbits and deep space missions.
It has 2,760 UltraScale DSP slices and provides up to 1.6 TeraMACs of signal processing compute, an increase of more than 10-fold, compared to the prior generation, said the company. To meet increased bandwidth, there are 32 high-speed transceivers (SerDes) that can run up to 12.5Gbps to deliver 400Gbps aggregate bandwidth. The FPGA provides 5.7 tops of peak INT8 performance, which is an increase of nearly x25 compared with the earlier generation, for deep learning.
The on-orbit reconfiguration, real time on-board processing and ML acceleration will allow satellites to update in real time, deliver video on demand and compute on-the-fly to process complex algorithms. As protocols and applications change, the FPGA’s adaptive compute architecture allows unlimited on-orbit reconfiguration before launch and while it is in orbit.
For ML development, the FPGA is supported by tools for TensorFlow and PyTorch frameworks to enable neural network inference acceleration for real-time on-board processing in space.
A ceramic package, measuring 40mm x 40mm is capable of withstanding vibrations during launch and radiation effects in orbit.
To accommodate the long design cycle, prototypes can be created before qualification and included in the board layout to be replaced by qualified parts to accelerate the design cycle, explained Sawant, reducing the design cycle to two to three years instead of five to six.
Programming the redesigned routing architecture with the company’s Vivado Design Suite can resolve common industry bottlenecks; it is claimed to eliminate routing congestiona and allow more than 90% of the device to be used without performance degradation. The company’s Vitis unified software platform can also be used for embedded software development on the triple modular redundant (TMR)-capable MicroBlaze soft processor. Future extensions will add support for Vitis AI, the unified software platform for AI inference on the company’s devices and production cards.
Flight units of the space-grade XQRKU060-1CNA1509 FPGA will be available in Xilinx Class B and Class Y test flows per the MIL-PRF-38535, from September 2020. Mechanical samples and prototype units are available now.