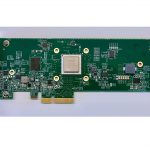
The InferX X1 edge inference accelerator is designed for processing real-time Mpixel vision workloads which requires high bandwidth support for deep learning models which operate with small batch sizes in real time. Typical workloads have deep networks with many feature maps and multiple operator types, explains the company. They may also have model accuracy targets which require the use of mixed precisions, including INT8, INT16 and BF16. The accelerator allows a mix between layers and is also designed for the low latency batch size (B=1 inference processing) typically required by these workloads.
The accelerator supports a x86 and Arm architectures and a choice of OS. It supports camera, IR, ultrasonic and RF sensor input types and Ethernet, USB and Wi-Fi comms standards.
The X1 dynamic tensor processor array is designed to support existing and future AI/ML models and is claimed to combine the speed and efficiency of an asic with reconfigurable control logic technology which futureproofs it by enabling the adoption and deployment of new inference model technologies via field updates. The accelerator architecture allows support for processing multiple data types including high resolution cameras.
In addition to the processor array’s MAC units and 12Mbyte of on-chip SRAM, the X1 architecture includes connectivity to external LPDDR4 DRAM for model weight, configuration and internal activation storage. There is also Gen3 /4 PCIe for connectivity to a host processor.
The company also offers the InferX edge inference software development kit with model compiler and runtime software. The model compiler converts models expressed in TensorFlow Lite or TorschScript and compiles them to operate directly on the X1 accelerator. The InferX Runtime controls the execution of the model and the X1 processes the data steam to generate the inference results.