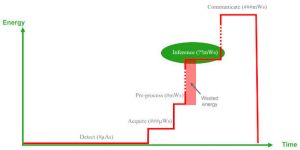
“Battery-operated sensors have, until now, been restricted to simple data sources such as temperature and humidity,” according to the firm. “But there is increasing interest in processing richer data sources such as images, sounds, and vibrations. These applications require significant processing on the edge device to remain compatible with low power IoT wireless networks such as LoRa, Sigfox, Narrow Band IoT, and Bluetooth Low Energy.”
It has been developed with Swiss research lab ETHZ and Italian reseach lab UNIBO – drawing from the PULP (parallel, ultra-low-power) project.
It has nine cores: one for control and an octa-core processing cluster.
“Multi-core allows lower clock and lower voltage operation so you get a quadrature power saving, but you have to parallel the applications effectively,” GreenWaves’ v-p business development Martin Croome said at the ‘Getting Started with RISC-V’ seminar in London last week. “We have seen a 7.7 to 7.8x speed-up on convolution and a 7.2 speed-up on FFT.”
To reduce power consumption, GreenWaves has taken up the option to extend the Risc-V instruction set to operate an on-die hardwired accelerator for convolution.
Why not hardware acceleration for other AI maths functions?
“AI is moving so fast – we don’t want to accelerate last years big idea,” said Croome.
Hardware and software development tools are available.
Applications are expected in people counting, object counting, image recognition, sound analysis, preventative maintenance and keyword spotting in wearables, smart toys, smart homes and roots.
Diagram: The challenge is to inference on a large volume of data, while
preserving typical MCU power behaviour.