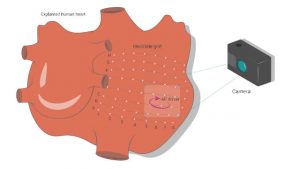
The team tested their approach on 11 donated human hearts and located AF drivers with an accuracy of up to 81%.
Multi-electrode mapping (MEM) is a technique that can be applied during an operation, in which an array of electrodes is pressed against tissue to measure electrical activity. But AF drivers have proved difficult to locate with sufficient reliability or accuracy using this technique – as the aim is to burn away the AF driver from within the heart tissue – called targeted ablation.
There is a technique that can accurately locate AF drivers, called sub-surface near-infrared optical mapping (NIOM), which has a resolution of 0.3mm, but is so invasive that it cannot be used inside someone during an operation.
The team posed itself the question: Could simultaneous MEM and NIOM data gathered from donated hearts be used to train a machine to accurately locate AF drivers using only MEM data.
And the results from this proof-of-concept study – that in 81% of cases MEM data alone would be able to guide ablation with sufficient accuracy – are promising.
“The trained ML model can pinpoint AF drivers on MEM, meaning it can potentially be used in clinic,” Alexander Zolotarev, PhD student at Skolkovo Institute of Science and Technology in Moscow, told Electronics Weekly.
“The data set of recording from 11 human hearts is both priceless and too small,” said fellow researcher Dmitry Dylov. “We realised that clinical translation would require a much larger sample size for representative sampling.”
For MEM measurements, two 8 x 8 electrode arrays were tried, one with 3mm pitch and one with 9mm, while cameras looked on to implement NIOM (see diagram).
The team at the 2019 Heart Rhythm Society conference
In training and use, signals from the electrodes were Fourier transformed into the frequency domain, then the heights and positions of frequency peaks were processed into data for learning or instantiation.
The 3mm array gave more useful results, but both had advantages.
“The general ML model combines the data from both MEM arrays,” said Zolotarev. “But only one of the arrays can be used during the mapping, they can’t be implemented simultaneously.”
‘Optical mapping-validated machine learning improves atrial fibrillation driver detection by multi-electrode mapping‘ describes the work in detail in the AHA journal Circulation: Arrhythmia and Electrophysiology. Only an abstract is freely available.
Skolkovo Institute of Science and Technology worked with Ohio State University Wexner Medical Center and the Russian Center of Computational and Data-Intensive Science and Engineering.
Diagram credit: Pavel Odinev Skoltech
Photo credit: Fedorov lab Ohio State