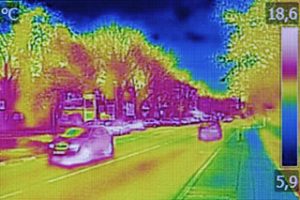
Infrared thermovision image showing lack of thermal insulation on Residential building and cars on the street
Why are automotive manufacturers interested in a technology that combines expensive, cumbersome thermal imaging and complex, computer-hungry AI to solve a straightforward problem such as pedestrian automatic emergency braking (PAEB)? The answer is because this combination works and nothing else does.
That’s not quite true, of course. Small, inexpensive RGB cameras combined with radar have been providing useful data to PAEB systems for several years, but only during the day. This limitation is hinted at in the test procedures provided by Euro-NCAP used by automotive manufacturers in Europe to evaluate new car safety. It includes night-time testing, but only above a minimum illumination that requires auxiliary lighting (streetlights). No testing is required under the unlit conditions found on the open highway or along country lanes or in many high-volume secondary thoroughfares.
US and European approaches
Rising pedestrian fatality rates, especially at night, are forcing a reconsideration of PAEB requirements after dark. In the US, the National Highway Traffic Safety Administration has initiated a process to establish mandated night-time testing for 2028- model year vehicles. In contrast, Euro-NCAP’s roadmap to 2030 is silent on mandates, preferring to award points for the presence of PAEB capabilities and other new protection systems. While several manufacturers are preparing to offer suitable equipment, in Europe the decision to select vehicles providing additional pedestrian safeguards will remain with the consumer.
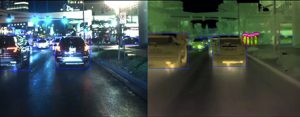
Figure 1: Thermal imaging permits pedestrians to be located even in chaotic scenes
What safety features will the consumer be able to select? Currently, even the best PAEB systems are successful only in the daytime under good visual conditions. They can be defeated by smoke, fog, rain and other phenomena that obscure the sight path. Bright lights, such as the sun setting or oncoming headlights, can mask the presence of pedestrians. Even clear, well-lit locations can be chaotic so that sorting out pedestrians visually becomes unreliable. Imaging pedestrians by their own thermal radiation can circumvent all of these degraded conditions because even in a cluttered scene, warm objects stand out to a camera that is sensitive to temperature variations.
Safety technologies
Radar is already used in some vehicles to measure distance. While performing admirably in many visually degraded situations, radar fails without support by camera data because it has almost no ability to locate objects in the scene. Distance information is useless without object positions.
Lidar is a newer proposed solution. Like radar it can determine distances accurately, but requires an active light source that is affected by the same visual obscurants that degrade camera performance. Lidar produces more data points than radar, but fewer than cameras. It is also expensive, with no obvious path to reducing cost sufficiently to make the technology affordable in vehicles.
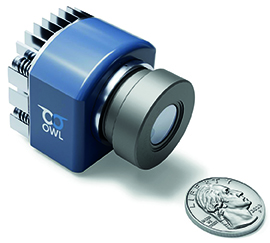
Figure 2: New design techniques produce small, light, rugged thermal cameras suitable for automotive use
Thermal imaging is passive. The radiation that forms the image is heat given off by any object, such as a pedestrian. No light has to be provided by the vehicle or any other source to be reflected by the object, so bright sources such as headlights or lasers are not needed.
Conveniently, thermal radiation penetrates most obscurants without significant scattering or absorption, so smoke and fog simply disappear. Furthermore, the sun and most artificial light sources contain little radiation that thermal cameras can see, so most bright lights also vanish.
Second, thermal imaging has been tested for more than 50 years in the most challenging of environments: battlefields. Thermal cameras have been flown, launched, dropped, and carried and survived. Making thermal cameras that will perform flawlessly in rugged vehicular installations is another adaptation of proven techniques.
Evaluating costs
Thermal cameras, especially models with sufficient resolution to meet PAEB requirements, have been expensive. Fortunately, the cost curve has been trending down for a long time, driven by volume applications such as building maintenance, security and firefighting. Acceptable prices will result directly from the volumes needed for automotive use, easily 1,000 times the size of the next biggest market. As production begins, the cost will ride down with the well-tested semiconductor volume curve.
Even so, thermal cameras will be more expensive than visual models so the number needed on a vehicle must be minimised. This is where AI can be employed. Finding distances with two cameras is straightforward through applying triangulation, but requires the cameras to be carefully aligned. AI works on images using a convolutional neural network (CNN) to identify items of interest in the scene. It compares new images with a large series of training images containing pictures of the objects of interest in all sorts of sizes and positions. Show a CNN a set of thermal images of pedestrians and it will flag things that look like these in any new images. This is not confined to objects as the images contain more subtle data such as disparity, which characterises how far the surface captured by a small group of pixels is from the camera.
Train a camera on known disparities and the CNN can produce a disparity map for the entire image. Find the objects of interest with one CNN, then label them with their disparities from another CNN and every pedestrian can be marked with the distance from the vehicle using a single camera.
To implement in a passenger vehicle, automotive electronics architectures have already begun to shift from the distributed model with hundreds of microprocessors, to the central networked model with a few very powerful computers capable of running all vehicle software for everything from passenger comfort to advanced driver assistance systems (ADAS). Ultimately, all the sensors in vehicles will rely on these computers to provide the intelligence.
With the implementation of the new automotive electronic architectures underway, the demands for pedestrian safety are constantly increasing,
Breaking the mould
To meet automotive performance and cost goals, thermal cameras must move past the traditional camera configuration. For example, most uncooled thermal cameras maintain calibration by the periodic actuation of a mechanical shutter that blocks the incoming radiation to create a dark reference image. In PAEB systems such periods of blindness are intolerable. Owl Autonomous Imaging uses a proprietary process to compute and apply dark correction without interrupting the data flow.
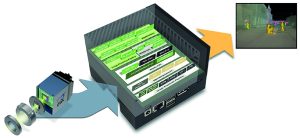
igure 3: A standalone system comprising a camera, processor and CNN software produces tagged thermal images in real time
The output at the end of this process is a digital data stream that preserves the small temperature differences the Owl sensor can detect. Most existing thermal cameras have analogue outputs, but digital data is a departure with data transmitted via high-speed serial protocols such as GMSL, which is an interface already in use for video transmission in vehicles.
Formatting the output of the thermal cameras to follow the output configuration of visible cameras eases the task of fusing the thermal images with data from other sensors to provide the maximum safety benefit from the entire sensor suite. Other sensors also use AI to extract information for various ADAS functions, so adding the thermal data does not require modification of the automotive data processing strategies.
Owl supplies CNN software suitable for automotive computing platforms and the image sets needed for training. To evaluate thermal imaging benefits even before vehicle systems integration, the ADAS development platform, a combination of camera, processor and software, acquires thermal images and extracts pedestrian location information.
With the availability of thermal imaging assured, automakers can incorporate this technology into new ADAS sensor suites as they improve the ability of automobiles to protect pedestrians at night.